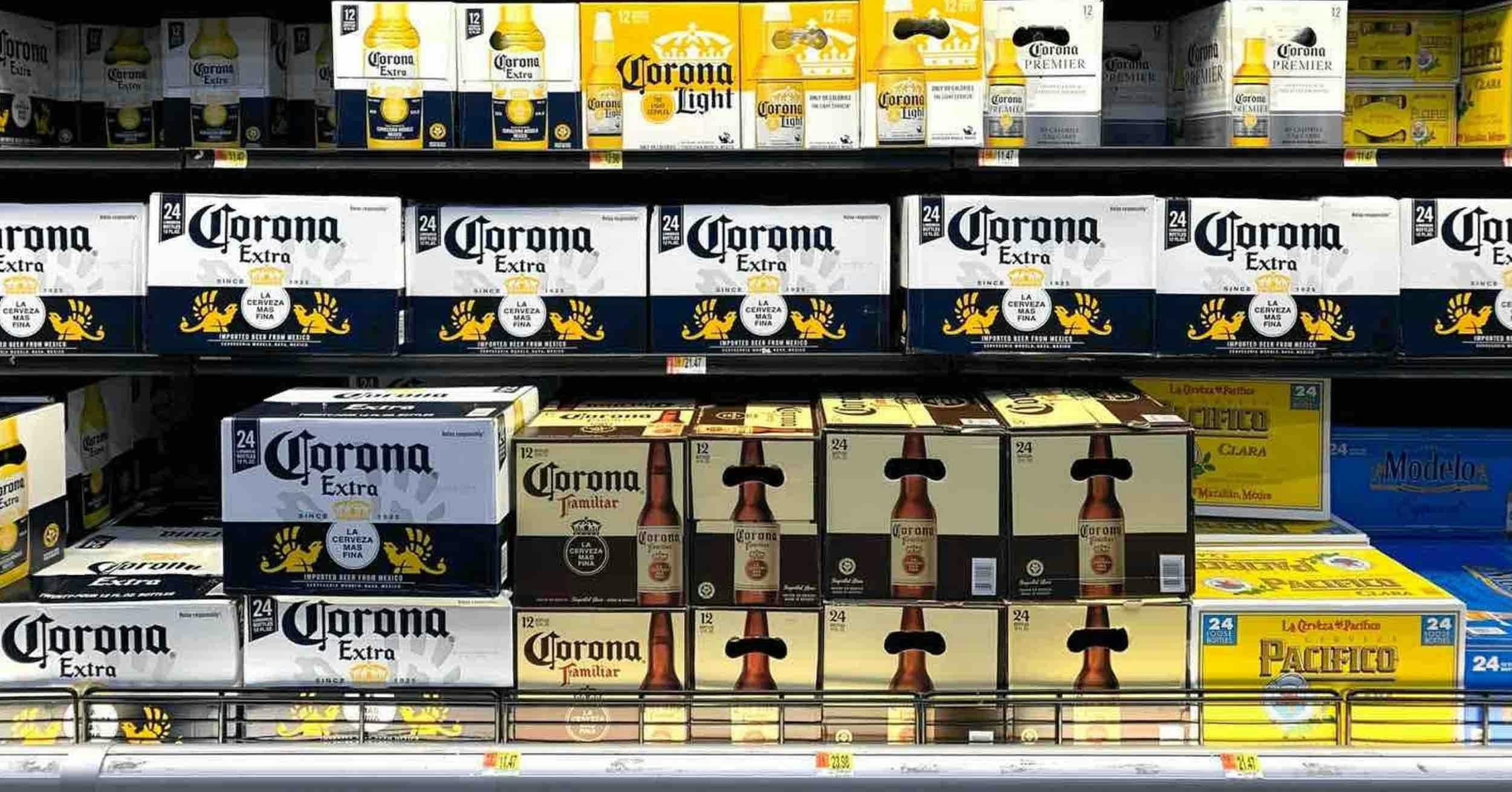
A five (5) min guide that will walk you through what you need to know about assortment, simulation, optimization & AI
Retail is a tough game, and optimizing your choices can be incredibly difficult. With the ever-growing number of SKUs to choose from, limited shelf space, and supply chain complexity, retailers and Consumer Packaged (CPG) companies are increasingly under pressure to get assortment right. Enter Artificial Intelligence (AI).
You can develop assortment strategies or review how your stores are doing, and hope for the best, but that may not give you the best results.
Question: Have you considered using computers to simulate and help you optimize your category assortments? Analytical assortment optimization powered by machine learning can greatly improve both decision and execution in rapid time. Here are a few key facts about this field and how you can use it in your category assortment planning.
What Is Machine Learning?
This is a segment of the field known as Artificial Intelligence (AI) and is designed to mimic the ways humans can learn things by importing data. While AI is a favorite topic in science fiction, the goal is to let computers improve their results through data and algorithms.
Machine learning (ML) is a subset of AI that uses special algorithms or models to conduct data analysis, finding patterns in data and relationships within the dataset, in order to make predictions. Predictions get better over time as more data is analyzed.
Trial and error plays a big part in ML. As the model finds errors or discrepancies between its prediction and the actual dataset, it tries to rectify its thinking so its predictions are close to what is actual. This process is often called "training the model". Actual datasets are split into training sets and validation sets, generally a 90/10 split with 90% for training and 10% for validation of its prediction or error rate. This is where data scientists might change the features of what the model should be learning from such as price, product, location and/or the model's parameters; which are the properties of the training datasets that are learned during training. Oftentimes, parameters are something that a model learns on its own and adjusts automatically as it tries to reduce its prediction error rate.
It is this ML trial and error that is important because it sees the world from a machine construct, not a human construct. This difference allows retailers and CPGs to tap into a new way of looking at, for example, assortment optimization.
Below is an illustration of this process of learning:
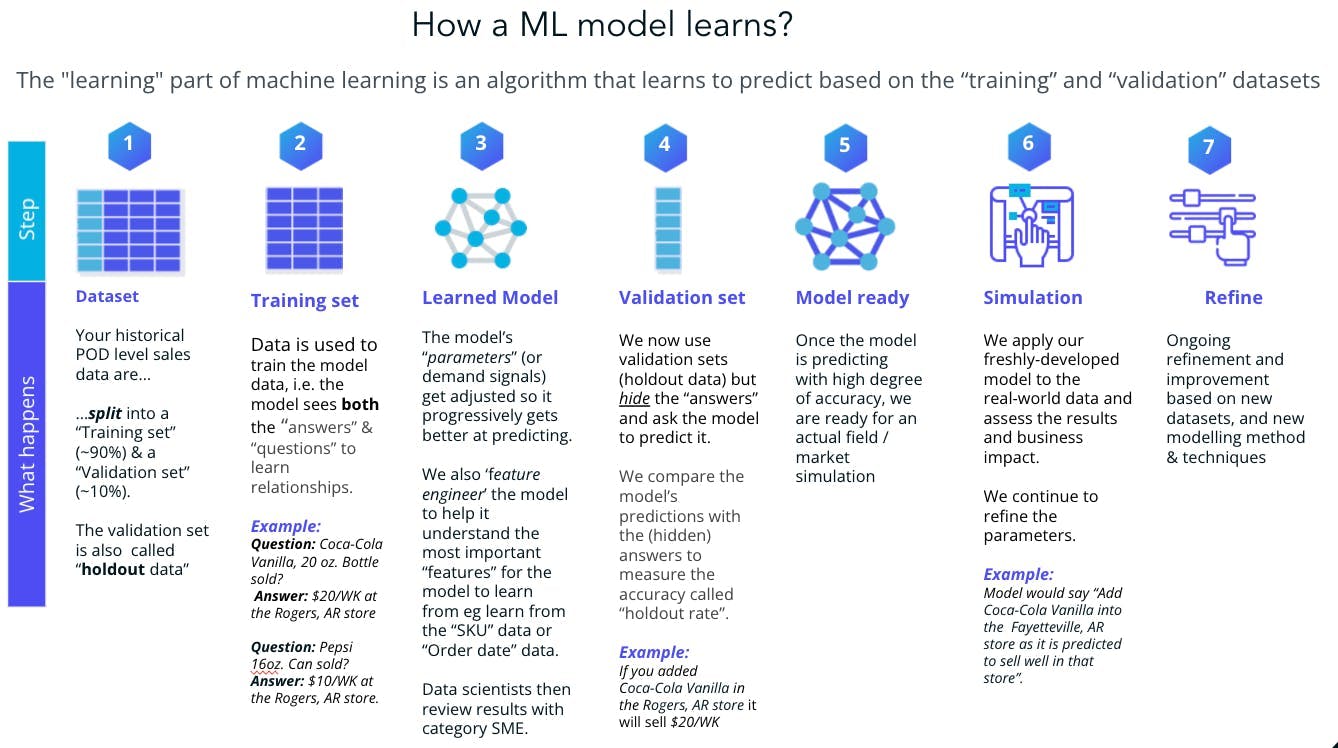
How Is It Used in Retail?
ML can help retailers optimize their supply, product, and sales. Retailers and CPG brands generate tons of data, and ML can look through this data to find new actionable insights for businesses to achieve their goals.
Many retailers use data analysis, and you've probably seen examples work in real-time when you're shopping or visiting a store. If you buy something online and get a "customers who liked this also like..." message, or if you see recommended items that match what you're shopping for, that's an example of a type of ML model.
However, there's also a lot of analysis and modelling that goes on behind the scenes. The location of stock on the shelves, and even what stock is in the store, can be improved with machine learning. Retailers and Consumer Packaged (CPG) companies can use ML models to find out where best to put items, how to shelve and advertise, and what things to restock. ML algorithms can effectively improve profit margin by ensuring a better product mix and uncover previously neglected customer needs. This means superior SKU rationalization and new product introduction as well as optimal space allocation and localization for those assortments.
Basically, the ML algorithms can be made to look at the data and help retailer/CPG assortment teams find out what moves stuff off the shelves. Do people buy a particular item more when it's on an endcap and is it worthwhile to have that item there instead of something else? ML models that are used for assortment optimization can perform core SKU analysis, letting you figure out your optimal SKU introduction for each store.
Some retailers also use ML to help them with loss prevention. If you've ever tried to buy something locked away in a special part of the store, you've seen this idea in action.
ML algorithms can be trained to pick items that are likely to be stolen. The stores then lock them up, cutting their losses on those stock items. While it can be frustrating, it serves as an important tool for keeping costs low.
What Can Analytical Assortment Optimization Do?
Analytical assortment optimization is a specific way to use machine learning to help you make decisions for your business. It can perform "retail simulation," giving you a virtual idea of the impact of your category or merchandising assortment decisions and recommendations on how to improve them.
Historical data is a key aspect of this kind of demand or assortment forecasting. ML algorithms will look at the sales and volume numbers from your entire universe of stores, trying to find patterns in the data. Did something sell really well in one store that can be sold in another? Which stores will this new SKU introduction do better in? The decision can be simulated rapidly and the impact quantified to inform decision making.
Again, more data is always better. However, the reality is there are also merchandising rules or constraints like POD (Point of Distribution) thresholds and ensuring certain SKUs get at least, say, 1.5 cases on the shelves. As you begin using analytical assortment optimization to track your sales and store's performance, you'll begin changing these constraints and be able to see the true impact of your decisions. As this happens, the algorithm will be able to better analyze what sort of changes are beneficial at store-level or national-wide.
How Can You Implement It?
There are a few ways to go about using data analysis and ML to improve your assortment decisions. If you feel confident about your statistics and programming skills, you can do the analysis on your own and try to improve your store.
But if you're like most people and barely know anything about statistics or programming, or simply don’t have time, you may have to seek outside help. Whether you want some planogram analysis, SKU rationalization, or retail optimization, it might be a good idea to pay someone else to help take care of things.
Tech companies offer data analysis on all types of business data. From your products to your advertising choices, the analysis can improve your profit margins and get your products moving. Everything from small things all the way up to the big picture with your macrospace allocation and localization can be covered.
It doesn't matter if it's big or small; data analysis can help you save money and improve your business!
Learn More
If you'd like to learn more about how analytical assortment optimization can help your business, we'd love to help! You can learn more about our solution, HIVERY Curate which enables you to run SKU rationalization, SKU introduction and optimization at store-level and up. HIVERY Curate can incorporate any merchandising constraints, allowing you to simulate strategic scenarios with accuracy and reliability, guaranteeing executable store-specific recommendations for every cluster dimension or count; eliminating the averages of averages approach.
Feel free to contact us with any questions or concerns about machine learning, AI, or data analysis you may have or just see a live demo. Seeing is believing!
Get this guide emailed?
Want this guide email to you for later reading? No problem, request it here