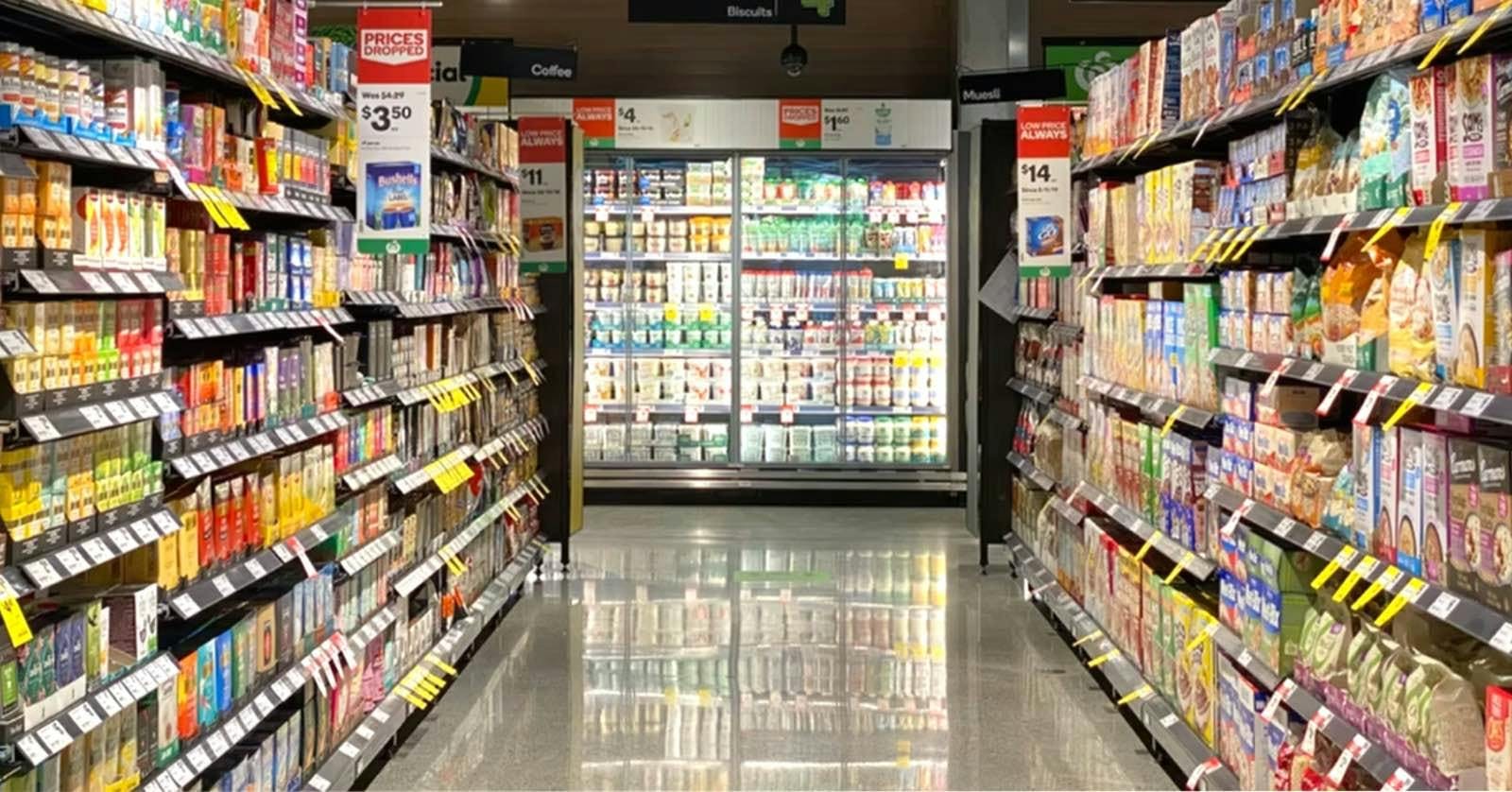
How AI-Driven Assortment Simulation Will Change Assortment Planning
How have the best assortment planning practices evolved? What can category analysts and shopper insight professionals learn to grow their professional skills in the new year? And how is AI shaping the future of category management?
Read on to discover how CatMan 3.0 will change every retailer’s approach to assortment planning on the Category Management Association (CMA). Then, learn how AI-driven assortment simulation ushers category management into the future.
Information in this piece is adapted from a podcast interview with Steven Soderlund, first featured on the Retail Maverick podcast. Soderlund is a CMA board adviser and analytical consultant for major retailers.
Category Management 3.0: How Category Management Has Evolved
Category management was first articulated as a practice around the 1980s. The original eight-step process outlined retailers’ and marketers’ supply and purchasing strategies for the next decade.
Then in 2016, customer insight professionals standardized some much-needed updates.
CatMan 2.0 incorporated new data sources, advances in analytics capabilities, increased retailer diversification, and greater shopper empowerment. Yet, for all the innovations, 2.0 still largely focused on brick-and-mortar retailers.
And, while 2.0 involved greater joint business planning among retailers and manufacturers, 2.0 did not deal with new fulfilment methods with the necessary complexity.
Enter: CatMan 3.0.
The latest formal category management process keeps much of what worked. Managers are still developing consumer insights and capturing information about shoppers. They’re still executing and iterating plans.
What’s changed? CatMan 3.0 incorporates full integration of the omnichannel mentality into the assortment planning process. It’s all about how to manage that change.
Omnichannel Retailing and CatMan 3.0
Omnichannel retailing harmonizes brick-and-mortar store and eCommerce services. It integrates marketing channels.
This, in turn, alters how analysts should conceive of shelf space in the store. In the past few years, online sales have grown to 15-20% from 5%, seemingly “overnight.”
In stores, it’s increasingly critical to dedicate shelf space to fulfil online orders. Same-day shipping and curbside pickup orders make an increasing bulk of sales.
New technology even lets customers peruse local stores' products from home. You don’t want bares shelves after items are picked up. Other technologies like Shopify enable more physical stores to go online in a manner of minutes.
CatMan 3.0 Impact on Category Analysts
CatMan 3.0 offers analysts a more robust list of metrics to track customer behavior. When following best practices, category managers ought to use the new, diverse metrics when they measure category performance.
The 3.0 edition also offers clearer, more detailed, more defined categories. This makes it easier to cultivate more nuanced decision sets.
Your customer decision tree will vary among similar categories: the customer won’t behave identically in the candy aisle in a store as they do browsing for chocolate online. Granular categorization lets you map out customer behavior more readily.
Categorize by Buyer Behavior: Brick-and-Mortar vs. Online
3.0 lets you increasingly define categories by consumer behavior and experience. Previously, you may only have used broad strokes to categorize products by behavior. For example, you might sort impulse purchases from routine/needs-based purchases.
Now, insight professionals can build categories around shopper patterns. A routine purchase, like laundry detergent, is better understood as customer experience in some contexts.
Is the customer buying detergent every eight weeks out of habit? Or are they moved to try experiential products with new scents?
In some cases, speed is not as paramount online, as online shopping is already convenient. Customers may be more willing to experiment or purchase something they saw mentioned recently on Facebook.
In contrast, displays in a brick-and-mortar store act as line-of-sight “billboards.” When you understand a customer’s patterns, you know which category displays more often alter behavior.
Takeaways for Category Managers and Shopper Insight Professionals
New categorization is possible because there’s more data available than ever. But how can you make data measurable and actionable?
The growth of omnichannel (i.e. eCommerce and direct-to-consumer) is an opportunity for all categories to grow. Retailers can meet shopper needs in new ways.
But, more complexities in the behaviors of consumers complicate things. For category management, it’s more critical than ever to measure shopper behavior. Then, it’s vital to apply measurements effectively.
Fortunately, there are new tools to do just that. AI-driven solutions make CatMan 3.0 work. With AI machine learning, category management will evolve past the spreadsheet.
Category analysts and managers will get in front of real people again. CatMan 3.0 empowers managers to do the fun stuff—like creative storytelling—while advanced AI solutions do the rest of the work.
Pandemic Upends Customer Purchasing Habits, Prediction Models
Why are AI solutions so critical?
Post-pandemic consumers are used to pandemic innovations. So many retailers rose to the challenge of the pandemic. Services like curbside pickup and same-day delivery are here to stay.
Now, a customer who's about to make dinner might choose a recipe on Pinterest. But, they realize they're missing an ingredient. That's not a problem—they can order that ingredient and it'll be at their door in an hour using services like Instacart.
Some customers may never return to brick-and-mortar stores. Others may split their time more evenly between online and physical shopping.
SKU Innovation, Big Data Change Demand Forecasting
Tech innovations have changed the game for category managers. CatMan 3.0 advises retailers on the best way to take these into account. When you build your approach for 2022, keep in mind:
- SKU innovation
- Multi-channel innovations
- Retail store options
- Changing product preferences
- Social media influences
- Growing datasets
- A growing list of retail and merchandising rules and constraints
These innovations factor into the customer behavior models of the future.
For example, SKU rationalization empowers retailers to uncover brand growth potential. As demand transfers, SKU rationalization creates space to swap-out underperforming products and reduce out-of-stocks. With AI, you can model different swap-out or churn rates and see the impact on revenue. Below is a real AI assortment simulation prepared for a client presentation. They wanted to know the break-even point of their assortment change (ie adding new items vs deleting old poor performing SKUs) at stores. Below you see it would require this client to change at least 13% of assortments to get to the break-even point. This was all powered by using HIVERY Curate and created in a matter of minutes, not days or months.
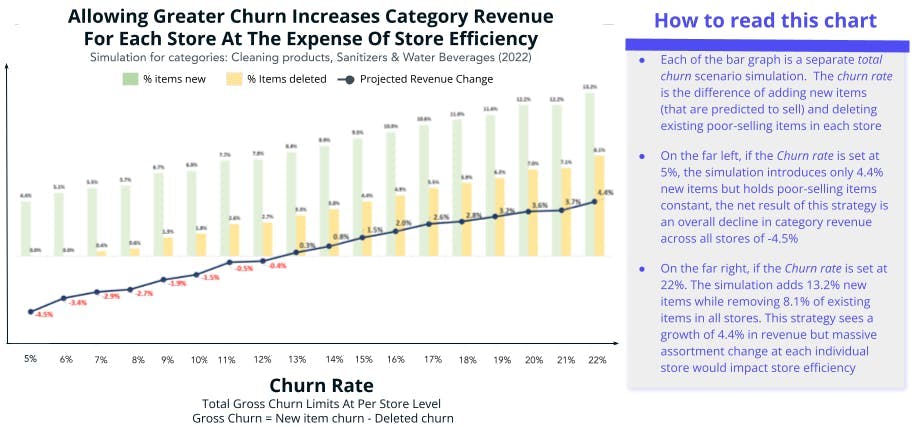
Further in the Curate solution, if they wanted, the Category analysts and shopper insight professionals can view are the actual assortments that are being deleted and new ones added. They can also view by individual stores.
Today any Category analysts and shopper insight professionals would need to upskill to manage this level of increased information complexity. With solutions like Curate that are powered by artificial intelligence, such analysis can be done in a few clicks. This means you can conduct any “what if” scenario planning at the store level and up prior to implementing any major resets. Artificial intelligence can do this category-by-category, retailer-by-retailer and manage all associated merchandising rules and assortment constraints with category goals. The aim is to improve product placement efficiency on the shelf.
The future is artificial intelligence (AI) and it's available today.
What’s the Best New Approach to Assortment Planning?
As retail changes, the available data broadens and merchandising rules/constraints will be complicated. The big data companies, Numerator, Nielsen and IRI, generate a lot of useful syndicated data. They also offer panel data, which can inform your consumer behavior maps.
Meanwhile, eCommerce data also grows. Clickstream and other solutions enable broad data collection in the eCommerce space.
You can't afford to ignore it. But, you must avoid drowning in the data.
The required legwork has only increased. You still need to discern the appropriate regional segmentation of assortments per retailer—but now you have more information to sort through.
What's the best way to pull the signal from the noise?
The answer is AI-driven solutions.
With AI-driven solutions, you can do the exercise that matters in minutes. Build a story with actionable insights.
How do AI and ML Solutions Make a Difference?
Ai-driven solutions get you to the actionable result faster. You can create more accurate predictions now than you ever could before.
A solution like HIVERY Curate turns big data into a sales forecast. But, it doesn't stop there. The AI program then takes granular data at the assortment level and cultivates an actionable plan that has been already qualified. Meaning you know the likelihood of your plan if executed in retail space. Curate has simulated the assortment plan's financial impact and included your category goals and those retail and merchandising rules and constraints.
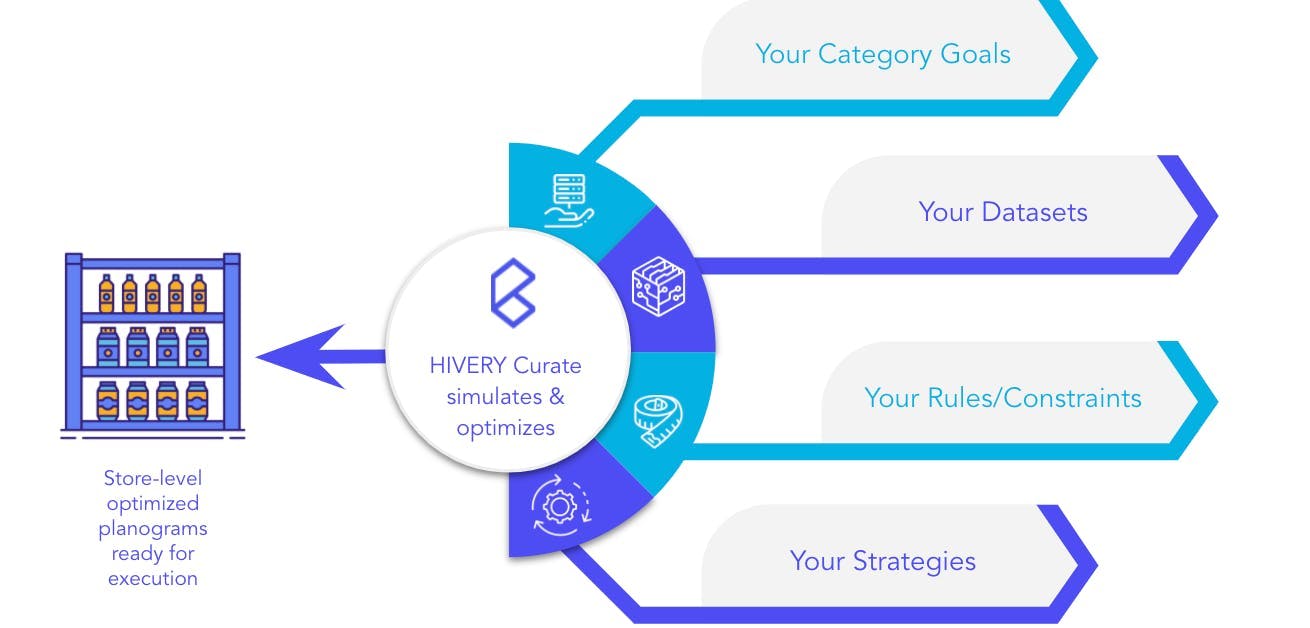
How Machine Learning Works to Make Assortment Predictions
HIVERY Curate optimizes assortment and space using big data. Typically that's retail data and POS data from all stores and all items.
HIVERY Curate incorporates omnichannel data into its recommendations. This includes all sales velocities as well as the category goal and merchandising rules and assortment constraints.
Once the AI looks at the data, it makes predictions: which products will sell in which environments? How quickly?
Thus, with Curate's plan, you can create the most appropriate amount of space for each category.
Test Hypotheses With Machine Learning
As we know machine learning is just a sub-area of AI. It's where algorithms learn unique patterns in data beyond human abilities. This means you can test category management strategies hypothetically not possible before
For instance, you could go into HIVERY Curate and ask “if I take out these 5 items, what will happen to this category?” or “What new items should I be adding in? What is the optimal assortment for these selected stores? What is the impact on sales of reducing distribution for a supplier? What happens to volume and sales if we deleted 18 & 20 pack bottles and cans? Where will the demand transfer to?
HIVERY Curate will run simulations and generate forecasts given your merchandising rules and assortment constraints or any other parameters that you like it to include.
HIVERY Curate's forecasts aren't guesswork. It is trained. The simplicity is that it does not need information like demographic or seasonality. The demographic and seasonality traits of each store are already “embedded” in the POS data (ie point of sale or point of purchase). Further, AI solutions like HIVERY Curate are trained to understand important economic principles like product cannibalisation, elastic of demand for each SKU and demand transference. So in a few clicks of the mouse, it delivers actionable recommendations with associated planograms should you want to execute that category reset.
With machine learning, you have a quick and easy way to test any assortment hypothesis. A predictive model no longer requires retail disruption, you just need to test the hypothesis and see the impact before.
How AI-Driven Solutions Apply Economic Concepts
As mentioned, AI-Driven solutions apply known economic concepts before they generate an actionable plan. For instance, HIVERY Curate specifically understands concepts like:
- Assortment elasticity
- Cannibalization
- Incrementality
- Demant transferrence
Consider item transferability vs. cannibalization. A marketing team may be too attached to brands to predict accurately. The team may be overly-optimistic about a new scented deodorant product within their brand.
Will the new product expand the brand's consumer base? Or will it simply cannibalize the brand's existing deodorant products?
An AI-driven solution takes a logical, mathematical approach to predict incrementality. It learns how economic rules have been applied before, then applies the most similar scenarios to the proposal.
Thus, the team gains a more accurate sense of whether the new product will upend the market or cannibalize sales.
Can Machine Learning Predict New Product Development Before Data Exists?
Yes, machine learning solutions can predict how customers will react to new products. A machine can also continually update its model based on new data.
Category managers can use HIVERY Curate to predict a new product's impact. To do this, a manager assigns a new product a "sister item." Curate then discerns relationships.
HIVERY Curate examines the sister product's relationships to other products in different categories and in different types of stores.
Then, the AI incorporates transferability. This empowers the AI to note the difference in relationships and contexts between the new item and the sister item.
This, plus input POS data, enables Curate to generate an accurate model of customer response to a product that's still in development. Alternatively, you might want to leverage market research data points like new product penetration rates from say a Nielsen BASES report. You can add percentage data and see the impact all at the store level.
AI-Driven Data Analysis, Human Creativity: CatMan 3.0
Assortment planning demands our due diligence. The future of category management requires us to use giant sets of data, those merchandising rules and assortment constraints. That makes tools like HIVERY Curate critical.
HIVERY Curate frees us from number-crunching tasks. With the data managed by artificial intelligence, we can do what humans do best: create stories.
Free the creativity of category managers everywhere. Explore Catman 3.0. Request a demo of HIVERY Curate today - the world’s first true store level strategy simulation & optimization solution.