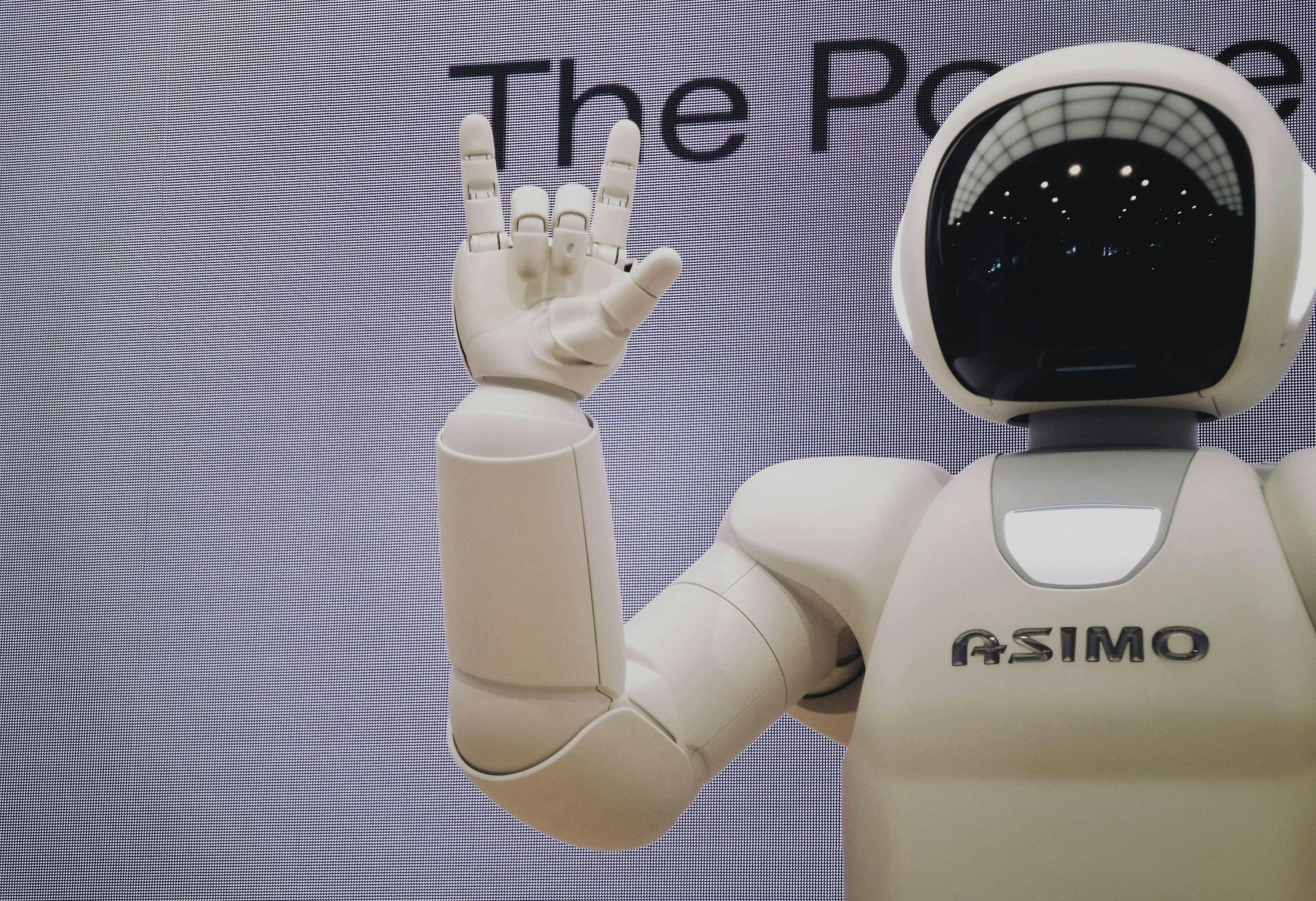
6 steps to roll out ai in your company
Get your business ready for the next industrial revolution.
AI such as Machine Learning is a comprehensive technology – you know that already.
But rolling out any technology, especially a new one, can be daunting for any company of any size. There are so many moving parts, so the project management takes longer and more business units are involved the larger a company gets.
As impossible as it seems, companies figure out how to do it every day. Here’s a quick six-step plan to get started in shifting your company towards implementing AI solutions.
To start, I think you should agree on a very high-level process. At HIVERY, for example, we have our Discovery → Experiment → Deployment approach. This approach has ensured that we consistently address new customers needs and design AI applications that learn over time to address key business goals. It requires us to continuously co-design and co-experiment with our customers leading to faster sales conversion while creating simple and easy-to-deploy AI applications.
With that in mind, let’s explore the six steps in getting your AI project underway:
1. Focus on the problem, processes, dataset, and business rules
Defining the business problems means understanding what you are trying to solve. You need to take time to define what you want the AI model (or algorithm) to do. AI is great at achieving goals.
Do you remember the movie I, Robot, and the AI called VIKI? Its goal was to protect humanity; however, VIKI soon realized humans’ greatest treat were other humans! VIKI came to the conclusion that the biggest threat to humanity is humanity itself, and the only way to protect humanity is to take control over it rather than protect it.
Defining the AI model’s goal is important. AI is good at accomplishing a goal. Check out this Google Deepmind video on learning and achieving goals. The AI algorithm knew nothing about the controls. It was fed raw pixel data and had to decide on the next action. It had no idea of the principle of a ball or the game itself. The only goal was to maximize the score.
Defining the problem also means understanding the datasets associated with the process, the systems that hold these datasets, and how you can automate this going forward as you feed your AI model with new data.
The quantity and quality (or “integrity”) of the datasets are also important. For example, if you want your AI model to recommend an ideal product assortment for an outlet such as a vending machine, you will need at least 12 months of sales data to train your model of the entire vending machine fleet.
The quality of data is important here too. For example, if your backend system says column A in a specific vending machine is Coke and column B is Fanta, your ERP systems need to say the same thing.
This step is also about understanding the “business rules” or the constraints. Business rules are about ensuring consistency, quality control, and market execution. Your AI model needs to know this so that it can make recommendations that are realistic and operational.
2. Get your language right
Before you start, it’s important that you have a clear set of common terminology. Sounds simple, but many times project teams and management have miscommunication and misalignment. Terms like mean absolute error (MAE) or mean absolute percentage error (MAPE), root-mean-square error (RMSE) or root-mean-square deviation (RMSD), prediction error (PE) often times are interchangeability used. Even terms like experiment vs. pilot vs. prototype vs. proof of concept are mixed up. Getting the right terms amongst your stakeholders help set expectations, including management. We often agree on this upfront and create a simple project dictionary which is often re-used in presentations and statements of work.
3. Shift your mindset toward experimental
Experimentation lets you generate results quickly and think forward. It’s important to think lean. Start with a small team and plan out your “experiment design” so that your experiment is bulletproof when you present the results. You do not want any of your stakeholders to “pick holes” in it and destroy your credibility and the AI initiative with it.
Experimentation is about testing, learning, and validated value. So, determine, align and agree on your KPIs.The goal here is to learn, get results quickly, and validate the value.
4. Provide senior management support
The only way to ensure that directives seep into the company culture is if senior management supports it. Everyone looks to the c-suite for direction, so remain composed and get everyone in line before announcing the proposed changes to the rest of the company.
Getting your terminology right, articulating the problem you’re going to solve and how you designed your experiment in an objective and transparent way is critical to getting that management’s support.
5. Reskill your people
Change is scary for everyone, and deciding to implement AI is a much different task than actually rolling it out. Your team needs to be agile and prepared to make sometimes subtle and other times drastic changes to their daily processes.
Foster a learning culture within your organization, and AI will just be another new processor “person” in the mix. Set up training sessions, provide training materials and job aids, get input, and involve everyone in the company in this new change to keep everything running smoothly throughout the transition.
6. Get operationally ready
Once you have everyone’s buy-in, it’s time to prepare. An event like the World Cup or Olympics takes proper planning and preparation to execute when the time comes. Your business is no different – setting up test environments, preparing IT for mass installations and updates, and adjusting everything will ensure that the transition goes smoothly once it’s live.
If everything looks solid on paper and your team is ready, it’s time to start the rollout process in phases so that no current productivity is lost.
This is when your project management and change management teams kick in and take over. It’s about operationalization and scale.
By following these steps, not only will your company be AI-ready, but it’ll also be ready for any technology the future throws at it.
—
Sources:
Traditional merchandising and planning is dead
AI is to POS what the POS was to cash registers
How AI is revolutionizing manufacturing
AI could spur global growth