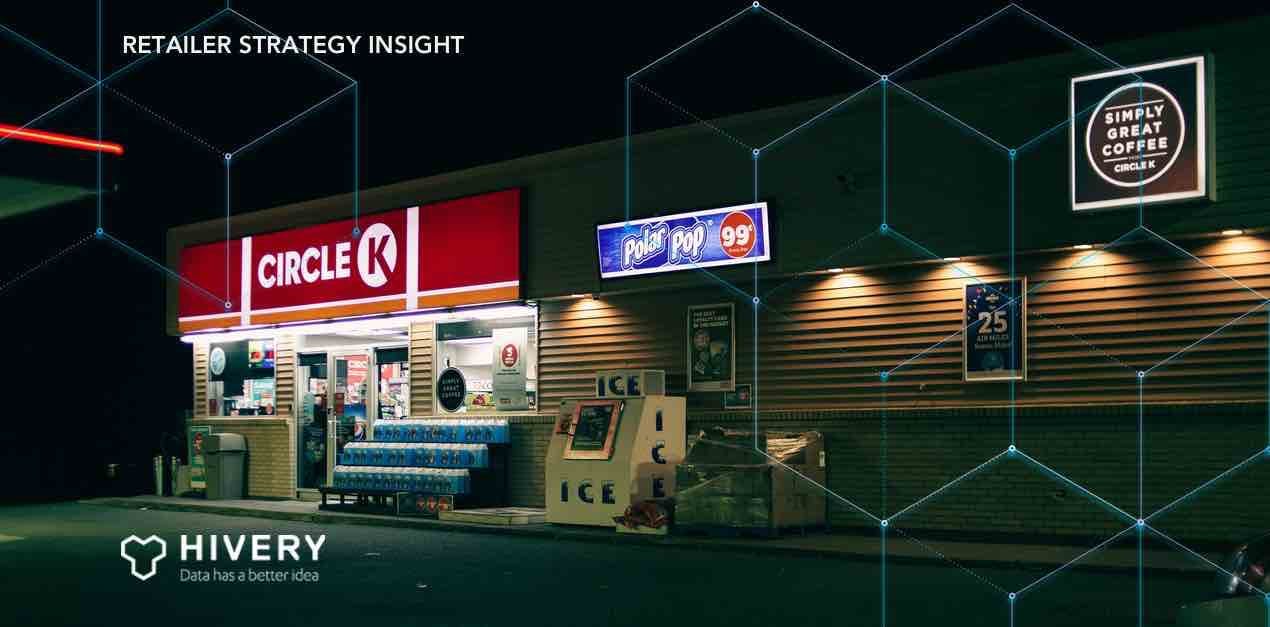
How AI Enables Convenience Stores to Be Community Stores Through Assortment Space Aware Planograms
RETAILER STRATEGY INSIGHT
Did you know that the average convenience store sees over 2000 customers daily? Interestingly, there are 150,000 stores in the USA alone, all competing for these customers' business. The average customer spends three to four minutes in the store. More importantly, a fair amount of the shoppers are local. In fact, according to the Association for Convenience & Fuel Retailing (NACS), 43% of Americas live within a mile of a convenience store (or “C-store”). In rural areas, almost 90% say they are within 10 minutes of a C-Store. These stores are often the only place to conveniently buy grocery items, fuel, or other products and services.
You have a perfect storm of precious local customers and many local stores trying to grab their attention. So how can advanced analytics, artificial intelligence (AI), more specifically, machine learning models enable convenience merchandising teams to be community stores? The answer: Augment their retail business expertise with the power of data and give local shoppers what they want and when they need it.
Today C-store retailers spend months strategizing, planning, and negotiating with hundreds of suppliers to determine the right SKU assortment and space mix for their stores. With AI, C-store teams can now rapidly find better business strategies to execute in each store by understanding the demand profiles of each store (or clusters of stores) and preparing assortment and space planning accordingly with suppliers. AI can simultaneously determine C-store’s SKU selection and space allocation at any cluster count you wish.
At HIVERY, we have taken years of data science research and partnered with leading data science organizations like CSIRO/Data61, designing and training machine learning algorithms with some of the world’s largest Point of sales (POS) datasets. Our optimized assortment and space recommendations are all inherently space aware and built from the store level up.
How AI Is Helping Convenience Stores
In recent years, AI has made significant advancements and is now utilized in various industries to automate tasks and improve efficiency. The retail industry is no exception, and AI is helping convenience stores become more community-focused.
The market today is very much divided between assortment tools and space tools. At HIVERY, we believe it is critical to consider demand and space constraints when planning for store shelf execution. HIVERY Curate leverages AI with applied mathematics (Operations research or “OR”) to unlock this ability and empower merchandising teams with the data science tools necessary to work at the scale of store-level sales and space data.
AI can help manage inventory levels and help with demand planning by providing dynamic insights into customer demand and learning from it. AI models discover relationships with simple datasets like point-of-sale (POS). These models rapidly assess, recommend, and deliver store-specific SKU selection, space allocation, and planograms at scale. While supermarkets average 15,000 to 60,000 SKUs in their stores, a typical convenience store has 500 to 1,500; getting localized assortment and space right is imperative.
When AI models learn from store-level data, they can discover relationships not possible with traditional tools or human abilities. By augmenting strategy with AI, you can rapidly find superior store-level recommendations that are space aware for any given category. With Curate, you can also run category strategy simulations, natively determine the financial impact of that strategy, and iterate for best outcomes. Once the AI model is trained, you can run unlimited different assortment planning or cluster design strategies. For example, what happens if I add the supplier’s new SKU innovation? What stores will it sell the best? What old SKU should I delete? What impact will that have on that category regarding revenue and volume? What is the impact on my Days of Supply (DOS) for each SKU for each of my stores?
How is this possible? Let’s review the following three (3) areas:
1. Bottoms Up Store Level Datasets
By leveraging store-level datasets, our AI looks at each store to understand what products are selling well and which are not and uncovers patterns across each store’s trading data. For example, it might learn one C-store in San Antonio, TX, has the same mathematical trading profile as another C-store in Philadelphia, PA. While humanly, we segment based on arbitrary segmentation principles such as demographic or geographical considerations, AI does not discriminate. It looks at relationships within the data, across all stores, and across all SKUs simultaneously. AI determines that a specific SKU sells well in the Pennsylvania store but is not stocked in the Texas store. Given that the AI learned that both stores have the same mathematical trading profile, it recommends a specific SKU for the Texas store. Something humans would not have ever considered.
Further, since it understands the individual store constraints, any assortment optimization applied is also inherently space-aware. It not only recommends that SKU for the Texas store but also decides the appropriate stock to supply it. This approach has several advantages:
- First, it ensures that the assortment is tailored to each individual store.
- Second, it reduces the amount of time needed to make decisions.
- Finally, it discovers incremental revenue opportunities not possible with current solutions.
In our experience working with 20 of the top 25 CPGs worldwide and in over 100 distinct categories, we have seen, on average, a 5% increase in incremental sales.
2. Rapid Strategy Simulation
Another approach that AI can take is rapid strategy simulation. Once an AI model is trained and learns each C-store's trading profiles, the planning process becomes more dynamic. You can interact with the model and run unlimited category assortment strategies. This approach delivers three key advantages:
- First, it is much faster than real-life testing strategies as it is done quickly and easily on a computer.
- Second, it is less risky as it allows experimentation without real-world consequences.
- Finally, it can provide insights that would not be possible to gain through real-world testing alone.
This means you can ask:
- How can I rationalize my suppliers and their SKUs to have the best assortment-space mix at each of my stores?
- What is the opportunity to re-optimize the existing cluster strategy?
- How can I perfect my days of supply strategy to reduce out-of-stock and food waste? AI identifies specific stores to distribute added items while assessing the impact of various assortment strategies on DOS. This avoids overstocking or understocking and reduces spoilage. We have a custom rule type that you can add to your strategy simulation called “Restrict Unsalable’ in a matter of seconds. For example, you can determine the DOS to reduce yogurt's expiration.
- What is the value of going store-specific? What stores will benefit the most? Since HIVERY Curate can identify unique demand profiles for each store, this allows store owners to design smarter cluster strategies and deploy capital wisely. By understanding data at the store level, we can re-grouped clusters with assortments and planogram counts that are optimized from the ground up.
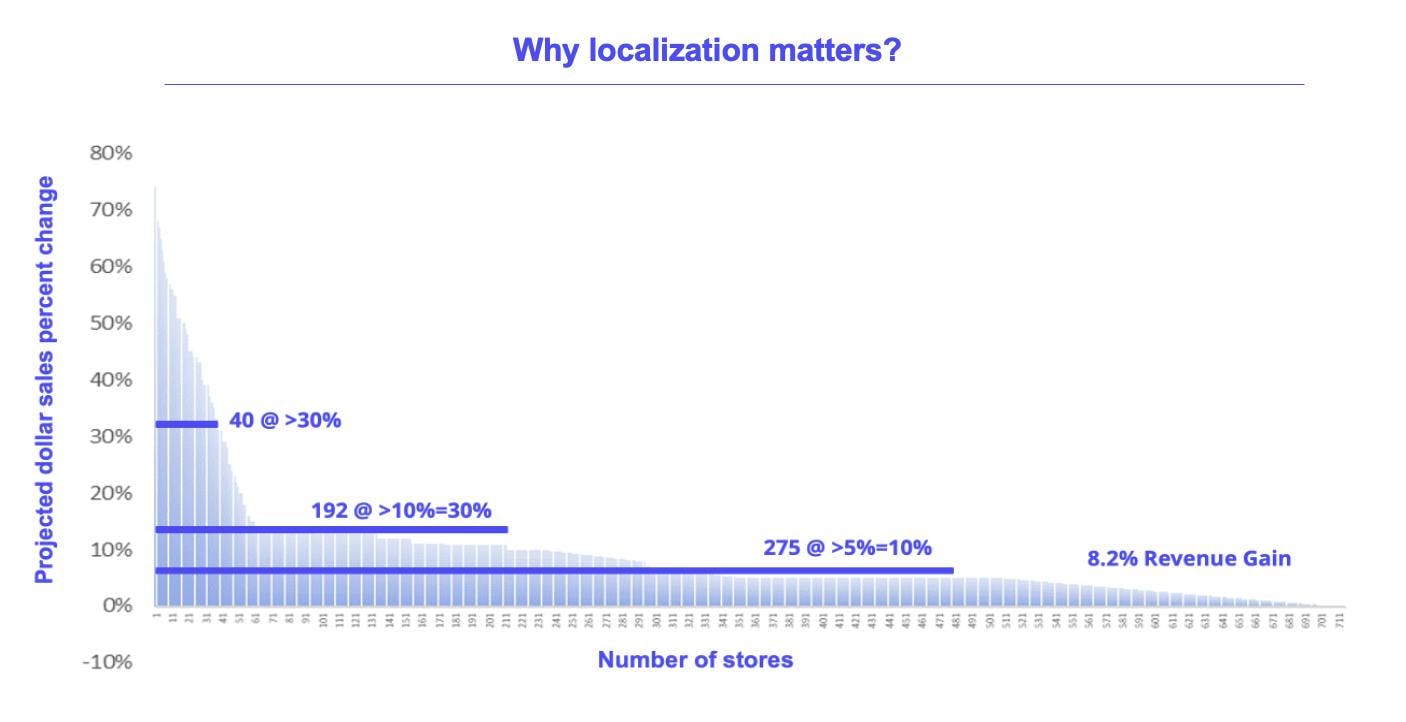
In the above chart, HIVERY Curate discovered new cluster strategies that were difficult to see using traditional methods. In this case, HIVERY Curate identified 40 specific stores with an opportunity to realize 30% ($30 of, say, a total of $100) sales opportunity. If they extend the recommendations to the next 192 stores, the opportunity increases to 60% (or $60). Going store-specific however allows you to maximize the incremental sales opportunities possible. The incredible part is that this analysis took a few minutes to generate, not months.
3. Guarantee Executable Planograms
After you have completed and agreed on your strategy, you can rapidly execute it. Since AI incorporates your own unique store goals and space constraints, any planogram generated will be executable on the shelf. These planograms can be exported as a PSA file from JDA/Blue Yonder for rapid execution across all your stores.
This approach means you are bridging the gap across strategy --> assortment --> execution ready from months to minutes. Again, from our experience working with top CPG brands and in over 100 categories, our customers have seen over 70% operational efficiency gain.
The Future of AI and Convenience Stores
With AI and OR, C-stores can offer a more personalized experience through the right assortment mix that helps build customer relationships and provides unprecedented operational efficiency gains.
The future of retail space management is here. Next-generation planogram space planning software solution leveraging AI and OR to offer a more “dynamic space planning” environment with strategically merchandise-aware planograms that are: locally relevant, effectively merchandised, operationally efficient, and allow for transparent retailer-supplier collaboration.
Are you ready to start optimizing your space with the right assortment planning? Request a demo today and find out how HIVERY Curate can benefit you.
Related resources you might be interested in:
Guide: Retail Store Cluster Strategies: Leveraging AI Towards Locally Relevant Shelves