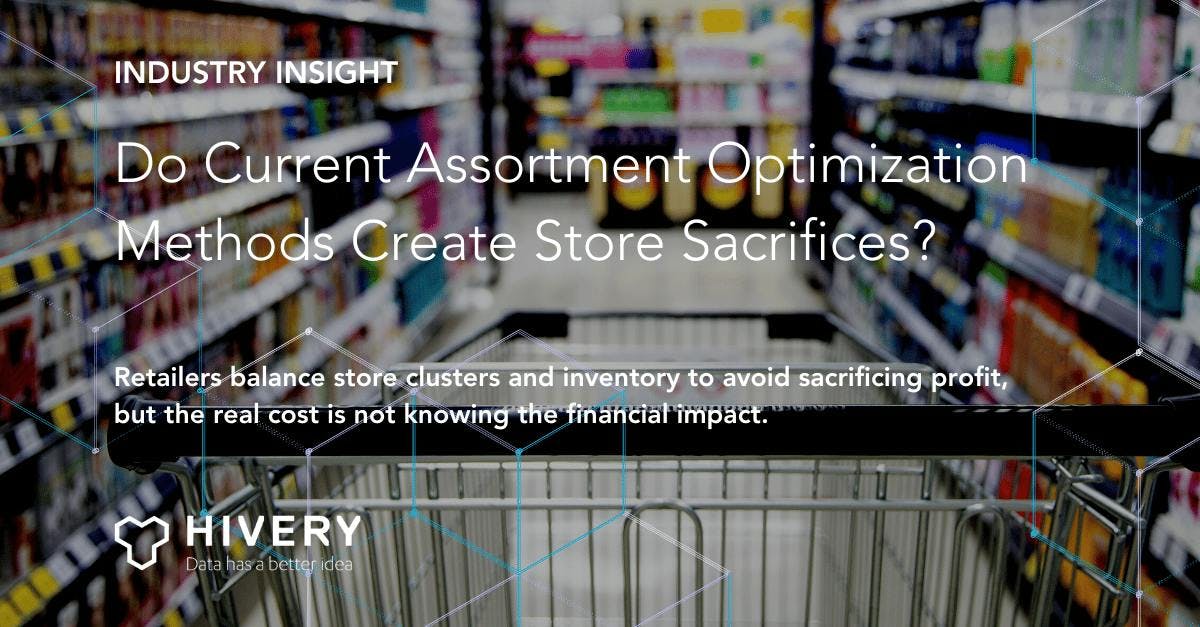
Do Current Assortment Optimization Methods Create Store Sacrifices?
Retailers balance planograms and inventory to avoid sacrificing profit, but the real cost is not knowing the financial impact.
Retailers are constantly striving to find and maintain the best selection of products to sell in their stores, which is a crucial part of their business. However, with a growing number of options, limited shelf space, store and neighborhood differences, and supply chain challenges, especially during the post-COVID-19 bullwhip effect, retailers face increased pressure to make the right choices.
With the rise of omnichannel, supermarket chains and retailers must ensure they have the right products in stock to meet customer demands and stand out from the competition. One solution to this problem is assortment optimization. According to a study by McKinsey & Company, retailers that use assortment optimization can see up to a 20% increase in sales and a 10% reduction in out-of-stock items. Additionally, it can lead to more efficient use of shelf space, allowing retailers to showcase more products and increase customer engagement.
Assortment optimization is a data-driven process that helps retailers make informed decisions about the products they carry in their stores. With the traditional method, the process of assortment optimization can be very complicated, time-consuming, and lacking real-world implications.
Why Traditional Assortment Optimization Methods Fall Short: The Sacrifice
Effective product assortment management and optimization involve constantly evaluating which items to remove, add, and introduce as new products. This process involves considering factors such as transferable demand and store-space availability. The process involves a continuous cycle of optimization and feedback, requiring significant data analysis, collaboration, and iteration. To streamline this process, retailers often group stores into clusters based on arbitrary variables such as store layout or proximity to the distribution center (DC). Putting, for example, 6000 stores into one cluster. Each of the 6000 stores will get the same assortment selection, and we know that operationally it might be effective. Still, intuitively, it is not desirable, at least not to the shopper who ultimately will miss out.
While this approach can make sense, it can also lead to many stores needing more inventory or less (out of stock and overstocking). Either way, creating unnecessary waste. Ultimately, retailers must balance the ability to prepare and execute planograms and perform complete category resets with the potential for excess or insufficient inventory at certain stores, which may result in sacrificing profit.
No More Assortment Optimization Sacrifice
When it comes to assortment optimization and sacrifice, data and a new way of using this data become critical. Companies must collect and analyze data on sales data, customer preferences, competitor offerings, and market trends to make the best decisions. Once the data has been collected, companies can use assortment optimization tools to analyze it and determine which products should be offered, where, and how much. With the use of AI-driven predictive analytics combined with “management science" (often called “Operations research”), retailers and suppliers can make more informed decisions when it comes to product assortment optimization without sacrificing profits. Such new methods enable rapid collaboration and the ability to run various scenarios, iterate with different cluster counts, and optimize clusters. Additionally, it allows for fine-tuning and optimizing the assortment strategy and comparing results at the national, cluster, or store level while considering those real-world constraints and rules in its modeling.
Fixing the Assortment Optimization Process
Assortment optimization is a critical component of retail success. It involves analyzing customer buying behavior and real-world constraints and deciding which products to carry in each store or store cluster. By optimizing the assortment of products, retailers can maximize their profits, increase customer satisfaction, and create a more enjoyable shopping experience from store operations, eliminating excess or out of stocks. New method in AI-driven tools opens up for much more dynamic approach to assortment optimization and planning. Far more collaborative and iterative. These new methods allow assortments to be more locally relevant, effectively merchandised, and operationally efficient.
HIVERY Curate provides retailers with an advanced assortment optimization solution. It combines machine learning, predictive analytics, and management science to give retailers insights into assortment plans. It also helps retailers identify gaps in their current product offerings and adjust their assortment accordingly.
In summary, the biggest cost, in reality, is not sacrificing per se but rather not knowing the financial impact of their assortment plans at the start. While demand forecasting and planning techniques help, it is oftentimes a blunt instrument. Such an instrument cannot cater to real-world constraints' data variety, granularity, speed, and specificity. AI and solutions like HIVERY Curate allows category management and retail buying teams to rapidly simulate unlimited assortment strategies while factoring in specific business rules and category goals to assess its financial impact.
Related resources you might be interested in:
6 Benefits Businesses Can Get from SKU Rationalization & How AI Can Accelerate them
Demand Sensing: The New Era of Retail Demand Planning
What Can We Learn About the Automotive Industry We Should Apply to Retail and CPG Industries?
Assortment strategies that generate smarter planograms with few resources