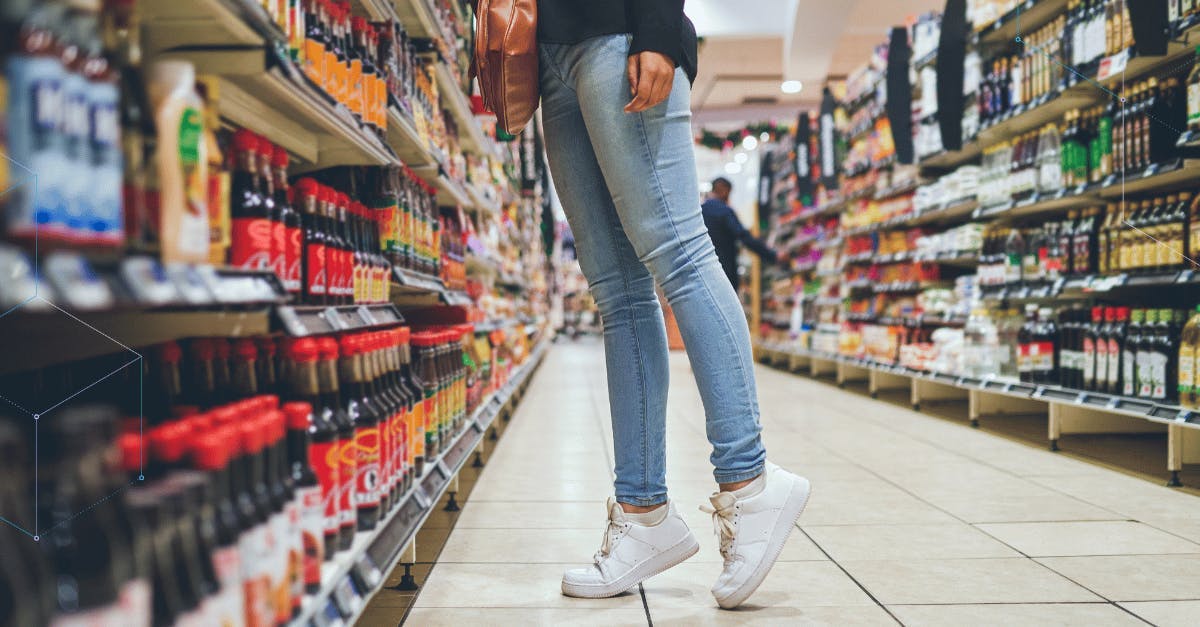
Deconstructing the Out-of-Stock Problem
In the complex world of retail, ensuring the right product is available to the customer at the right time is paramount. However, occurrences of out-of-stock (OOS) pose a significant challenge in achieving this goal. There are two sides to the stock problem, as pictured before:
- Store-specific OOS
- Shelf-specific OOS
Solving OOS can be challenging for both sides, but AI has the potential to offer assistance to both.
Let's dive into each and explore the causes of OOS and how AI can address these issues by extracting insights from store-level data and making informed predictions and decisions.
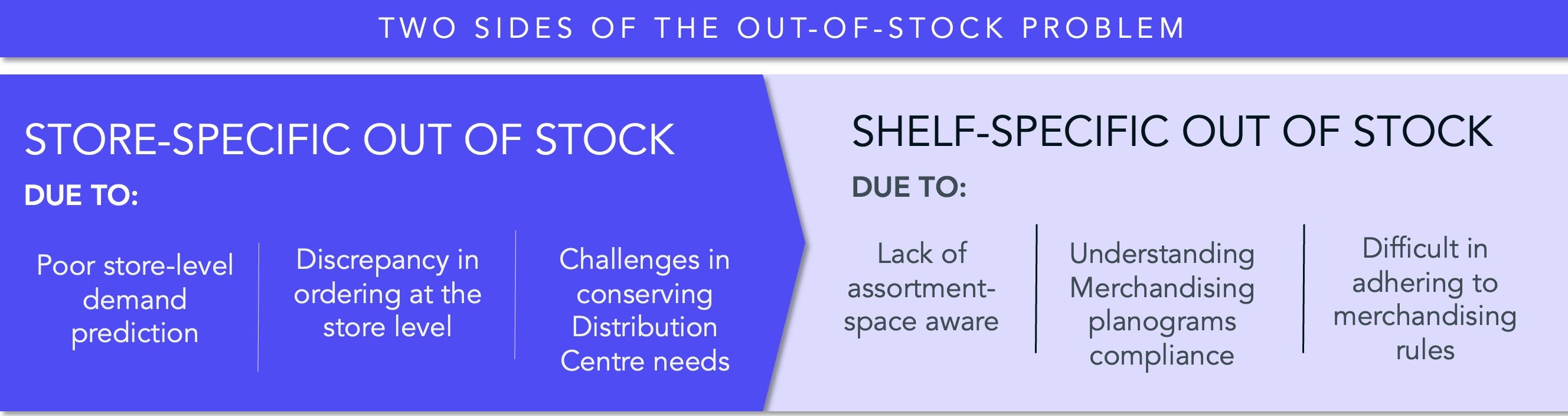
1. Poor store-level demand prediction
One of the primary causes of OOS is inaccurate demand planning. When a product is out of stock, it distorts the actual demand data, leading to underestimations and misinterpretations of sales and demand forecasts. AI can help extract insights from retail-specific store-level data, aiding in better-predicting demand and overcoming this challenge.
2. Discrepancy in ordering at the store level
The balance of products in the system often differs from the actual stock in the store, leading to inaccurate orders. Studies show that the level of coincidence is only 35-45%. By leveraging AI, the category management team can help minimize such discrepancies by providing a more accurate representation of in-store stock, reducing the likelihood of OOS situations.
3. Challenges in conserving Distribution Centre (DC) needs
Unsynchronized order and delivery schedules can also contribute to OOS situations. This often happens when the order is placed before the arrival of the early order. AI today can consider DC constraints in its modeling at the store level, ensuring more efficient and effective distribution, thus preventing OOS scenarios.
4. Lack of assortment-space aware
Shelf space allocation is another critical factor that can lead to OOS situations. Fast-moving goods may receive too little space, leading to frequent stockouts. AI models analyze store-level data to understand the store's latent shoppers and use AI to write rules into the model, such as "Force-in" specific SKUs at specific stores, ensuring better space utilization. You can read more about latent shoppers in this blog: "Uncovering Latent Shoppers: Bridging the Gap Between Stated Preferences and Actual Shopper Behavior."
5. Understanding Merchandising planograms compliance
Non-compliance with planograms can result in OOS situations. AI can apply rules to each store, such as "Limit Churn" to 5% when preparing a planogram. This helps ensure planograms are executed faster, impacting the operating costs of each store. You can watch this webinar, where we talk about how AI can qualify and optimize merchandising to any churn limit. Go to timestamp 13:30 mins here: [insert webinar link].
6. Difficult in adhering to merchandising rules
On-shelf item management is crucial for reducing OOS situations. AI models can consider an array of national, regional, store-level, or even SKU-level constraints. These constraints can include understanding on-shelf availability or ensuring heavy items are placed at the bottom, handling different types of store-specific fixtures (e.g., bunkers, capping, peg bars, gondolas), and merchandising styles (e.g., trays, cases, capping, nesting). AI can ensure adherence to merchandising rules, reducing the likelihood of OOS situations.
AI can extract insights from store-level data, allowing for more accurate demand prediction, efficient order placement, effective distribution, better shelf-space utilization, and more compliant planograms. This reduces the likelihood of OOS situations, ensuring that shoppers can always find the products they need when they need them. This not only increases customer satisfaction but also boosts retail success.
The real magic solving OOS: Optimizing Days of Supply
The real magic of how AI addresses OOS is by simultaneously considering the above six areas while optimizing Days of Supply (DOS). AI can reduce DOS through accurate analysis and optimizing the standard deviation reduction of DOS. AI can help reduce the variation or spread of DOS at the store level. You can read more here: "Optimizing Days of Supply with AI: A Closer Look at Standard Deviation Reduction."
If you want to decode your store's OOS, connect with our team of experts and explore the benefits of AI by using HIVERY Curate as your co-pilot.
Related resources you might be interested in:
- Can you find individual stores that are worth going to store-specific assortments? AI can
- AI's Potential to Empower Every Business: From Pizzerias to T-Shirt Store
- How to validate shopper assumptions & find new growth strategies for your retailer
- HIVERY's AI uncovers latent shopper insights & gains industry recognition